7 Our Problems In Data
Learning Objectives
In this chapter, you will
- Consider how data visualization can help you tell an informed, accessible story about your social problem.
- Apply thematic analysis to your interview with a class expert.
“Today, the average schoolchild aged between eight and eighteen years spends a third of her life sleeping, a third at school, and a third engrossed in new media, from smartphones and tablets to TVs and laptops. She spends more time communicating through screens than she does with other people directly, face-to-face” (Alter 2017: 237).
“The increased prevalence of Internet blogs and Web sites makes it easier and easier for individuals to engage only viewpoints they already share and to avoid unwelcome information at the same time. In essence, people are able to find information that serves and reinforces what they already believe” (Meyer 2015: 131).
When The Social Dilemma aired on Netflix in 2020, it pulled back the curtain on how media platforms leverage addictive algorithms to keep us hooked, serving up a constant stream of content that reinforces existing beliefs. Platforms like YouTube, TikTok, and Netflix profit from our consumption by recommending content based on our browsing history – content that keeps us engaged, but not necessarily informed. Overdramatic, sensational content delivers steady hits of dopamine, but it often fails to challenge our assumptions, introduce new perspectives, or present data-driven insights that complicate narratives about social problems.
In this landscape, it’s easy to retreat into ideological bubbles where two people with different browser histories are fed two completely different versions of reality. How do we resist this pull toward social silos? How do we break free from narratives that merely confirm what we already think we know?
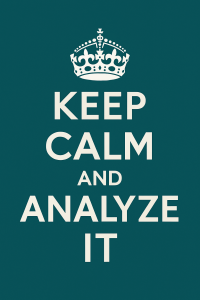
Data – whether quantitative or qualitative – provides a powerful means of moving beyond surface-level assumptions and uncovering underlying patterns and trends. By analyzing both numbers and narratives, we can tell more complete, compelling stories about what’s happening and why.
In this chapter, we’ll explore two complementary approaches to analyzing data: quantitative analysis through data visualization and qualitative analysis through thematic coding. As you engage with both methods, consider how each one offers unique insights while also intersecting to create a fuller understanding of social problems. By seeking out diverse data and analyzing it thoughtfully, we can gain a clearer, more nuanced understanding of the social problems that dominate our screens – and our minds.
Making Quantitative Data Speak
In a world flooded with information, data visualization can help us make sense of complex social problems by transforming raw data into compelling visual narratives that quickly convey key information. As the saying goes, “A picture is worth a thousand words.” When done effectively, data visualization can speak volumes.
The website Our World in Data is a powerful resource for exploring open-access datasets on some of humanity’s greatest challenges, from poverty and pollution to education and economic inequality. Max Roser, the site’s founder, believes that studying data on global challenges – and the progress we’ve made in tackling them – can offer valuable insights. Hear his take on a data-driven approach to social problems in the video Dr. Max Roser: Our World in Data.
To learn more about your expert topic, you will search Our World in Data for a visualization that illustrates a key aspect of the social problem you are studying. As you browse the data, consider these questions:
- What does this visualization reveal about the scale of the problem?
- What progress, if any, has been made?
- Whose experiences or perspectives might be missing from the data?
Data skills are in high demand across professional settings, where the ability to tell compelling, data-driven stories is increasingly valued. By leveraging tools like Our World in Data, you can practice distilling complex information into visuals that convey clear, impactful messages – messages that might just have the power to change how people see the world.
From Analysis to Impact
To tell a compelling story with data, we need to present it in ways that are clear, engaging, and accessible. Data visualization is the bridge between raw data and meaningful insights, allowing us to communicate complex information through visual means.
As you browse the vast collection of visualizations available on Our World in Data, your goal is to identify a visualization that effectively follows best practices in data communication. Learn more in the video, Telling Data Stories.
Before selecting a visualization, consider these key questions:
- What is the purpose of the visualization? Is it highlighting a trend, comparing categories, or showing a distribution?
- Who is the intended audience? What background information do they likely have? What questions might they bring to the data?
- What is the main takeaway? What does the visualization make clear? What might still be unclear or missing?
As you evaluate visualizations, look for evidence of the following best practices.
Chart Selection
- Does the chart type effectively convey the intended message?
- Histograms for distributions and frequencies
- Bar charts for group comparisons
- Line charts for trends over time
- Scatter plots for relationships between variables
- Pie charts and stacked bar charts for parts of a whole
Legibility
- Are labels oriented horizontally and clearly readable?
- Is the font size large enough to be easily seen?
- Are axis labels and data points clearly identified?
Organization and Simplification
- Are data presented logically, such as from largest to smallest?
- Is complex information broken down into manageable parts?
Color Choice and Accessibility
- Does the color palette include a high-contrast, color-blind safe scheme?
- Are colors used consistently and meaningfully to distinguish data?
Read more about accessible color palettes in the article “How to choose colors for data visualizations.”
As you explore the visualizations related to your expert topic on Our World in Data, consider why some are more effective than others. How do color, layout, and labeling influence your understanding of the data? How might the visualization be improved to align more closely with these best practices?
Data visualization isn’t just about making data look good – it’s about making data make sense. Pay attention to how effectively each visualization communicates its message, and consider how these principles can help you present information about your social problem clearly in your own work.
Highlighting Key Themes in Qualitative Data
While quantitative data can reveal broad patterns and trends, qualitative data analysis digs deeper to uncover the meanings, perspectives, and experiences behind the numbers. After interviewing a classmate about their expert topic, you will need to analyze the transcript of your conversation to identify key themes.
Thematic analysis is a widely used method of analyzing qualitative data. As Braun and Clark (2006) explain, it is “a method for identifying, analyzing, and reporting patterns (themes) within data” (79). This approach helps condense large volumes of text into manageable chunks while highlighting key insights and recurring patterns (Sheppard 2020).
Conducting thematic analysis typically follows a structured yet flexible approach, with early analysis producing insights that are integrated into subsequent rounds of review. In this way, thematic analysis builds insights as it progresses, like delicious layers on a data cake. The following steps offer general guidance for analyzing your interview transcript.
Immersing in the Data
Before identifying themes, you need to become deeply familiar with your transcript. This step involved multiple, attentive readings to identify initial patterns and impressions. You might highlight phrases that seem significant, note recurring words, or jot down initial observations.
Example: In a transcript of an interview about environmental pollution, you might notice repeated references to “worry about health” or “lack of clean water.”
Coding the Data
Coding is the process of assigning labels to segments of text that represent specific ideas or concepts (Sheppard 2020). Think of coding as adding hashtags to your data. Just as a hashtag (#Laker4Life) links related social media posts, a code links related segments of text.
Example: You might use the code “Health Concern” to tag any reference to health issues caused by environmental pollution.
Learn more in the video, What Does Coding Look Like?
Identifying Themes
After coding the data, you will begin to group similar codes together into broader themes. Themes are conceptual categories that summarize what the data is about. According to Saldaña (2016), a theme is “an extended phrase or sentence that identifies what a unit of data is about and/or what it means” (199).
Example: If you have multiple codes related to concerns about water quality, access to clean drinking water, and health impacts, these could be consolidated into a broader theme, such as “health impacts of water pollution.”
Refining Themes
After identifying themes, it’s time to refine them. This step involves reviewing the data to assess the clarity and distinctiveness of each theme. Look for overlapping themes, vague themes, or frequently occurring codes that could be split into subcategories.
Example: If the theme “health impacts of water pollution” includes several references to both physical health (e.g., illness) and emotional health (e.g., anxiety), consider whether these aspects should be presented as separate themes.
Reporting Themes
The final step in thematic analysis is to report the themes clearly and coherently. This involves writing a narrative that explains the significance of each theme and how it relates to your research question. Include illustrative quotes from the data to support each theme.
Example: My interviewee frequently expressed concerns about the impact of water pollution on health, stating, “People are afraid to drink the water, but we have no choice.” This theme underscores the intersection between environmental degradation and community well-being.
Qualitative data analysis involves intensive, repeated review of transcriptions. It is a lengthy process, but it’s important to give it the time that it takes. The more a researcher interacts with their data – during collection, transcription, and multiple rounds of analysis – the deeper their understanding of conceptual themes grows.
Using a qualitative software program to analyze data on a computer can make the process more efficient. Codes can be recalled for review and comparison on a screen together and coded data can be exported for storage and use in reporting. Many software programs exist, but Taguette is particularly useful for entry-level coding because it is simple to operate, freely available online, and contains thorough user guides with operating instructions. Follow the link at right to get tagging!
In Sum
As you complete both the Visualizing Problems and Interview Themes assignments, consider how data visualization and thematic analysis intersect. What does each form of analysis reveal that the other might overlook? How might combining quantitative and qualitative data offer a more complete picture of social problems?
Data analysis is more that a technical skill – it’s a powerful tool for telling stories that matter. By integrating numbers and narratives, we can gain deeper insights into social problems and present findings in a way that informs our audience rather than strictly alarming them.
References
Alter, Adam. 2017. Irresistible: The Rise of Addictive Technology and the Business of Keeping Us Hooked. New York: Penguin Press.
Braun, Virginia and Victoria Clark. 2006. “Using Thematic Analysis in Psychology.” Qualitative Research in Psychology 3(2): 77-101.
Gates, Bill. Dr. Max Roser: Our World in Data. YouTube. Accessed 16 May 2025 (https://youtu.be/HQSQ16dntbc?si=asoOC0Dp5bk-smP8).
Gibbs, Graham and Celia Taylor. 2010. “How and What to Code.” Online QDA Accessed 16 May 2025 (http://www.acrn.eu/cambridge/downloads/files/How%20and%20what%20to%20code.pdf).
Meyer, David S. 2015. The Politics of Protest: Social Movements in America. New York: Oxford University Press.
Mod.U. 2017. What Does Coding Look Like? YouTube. Accessed 16 May 2025 (https://youtu.be/phXssQBCDls).
NullQueries. 2021. Telling Data Stories. YouTube. Accessed 16 May 2025 (https://youtu.be/AD6-iDuoQFQ?si=rePtgAvjnlVhZIOG).
Orlowski, Jeff, Larissa Rhodes, and Vickie Curtis. 2020. The Social Dilemma. Netflix. (https://www.netflix.com/title/81254224).
Saldaña, Johnny. 2016. The Coding Manual for Qualitative Researchers. Los Angeles, CA: Sage.
Sheppard, Valerie. 2020. Research Methods for the Social Sciences: An Introduction. Pressbooks CC-NC-SA 4.0. Accessed 16 May 2025 (https://pressbooks.bccampus.ca/jibcresearchmethods/).
Tableau. 2025 “Visual Analysis Best Practices: A Guidebook.” Salesforce, Inc. Accessed 16 May 2025 (https://www.tableau.com/learn/whitepapers/tableau-visual-guidebook).
Yi, Mike. 2025. “How to Choose Colors for Data Visualizations.” Atlassian. Accessed 16 May 2025 (https://www.atlassian.com/data/charts/how-to-choose-colors-data-visualization).
Data expressed as numbers.
Data expressed as text or images.
An accessible way to understand data by representing trends and patterns in graphics.
The intended viewers of a data visualization who will use the information communicated for a specific purpose.
The ease with which a viewer can interpret a text or visualization.
A color scheme used in data visualization.
An exact record of what the interviewer and participant said in an interview, word-for-word.
The process of marking a section of text with a label that can be recalled be a software program (Gibbs and Taylor 2010).
A short word or phrase that sums up or explains what a section of text means.
Categories summarizing a prevalent topic in the data.